python里反向傳播算法詳解
反向傳播的目的是計算成本函數C對網絡中任意w或b的偏導數。一旦我們有了這些偏導數,我們將通過一些常數 α的乘積和該數量相對于成本函數的偏導數來更新網絡中的權重和偏差。這是流行的梯度下降算法。而偏導數給出了最大上升的方向。因此,關于反向傳播算法,我們繼續查看下文。
我們向相反的方向邁出了一小步——最大下降的方向,也就是將我們帶到成本函數的局部最小值的方向。
圖示演示:
反向傳播算法中Sigmoid函數代碼演示:
# 實現 sigmoid 函數return 1 / (1 + np.exp(-x))def sigmoid_derivative(x):# sigmoid 導數的計算return sigmoid(x)*(1-sigmoid(x))
反向傳播算法中ReLU 函數導數函數代碼演示:
def relu_derivative(x): # ReLU 函數的導數d = np.array(x, copy=True) # 用于保存梯度的張量d[x < 0] = 0 # 元素為負的導數為 0d[x >= 0] = 1 # 元素為正的導數為 1return d
實例擴展:
BP反向傳播算法Python簡單實現
import numpy as np# 'pd' 偏導def sigmoid(x): return 1 / (1 + np.exp(-x))def sigmoidDerivationx(y): return y * (1 - y)if __name__ == '__main__': #初始化 bias = [0.35, 0.60] weight = [0.15, 0.2, 0.25, 0.3, 0.4, 0.45, 0.5, 0.55] output_layer_weights = [0.4, 0.45, 0.5, 0.55] i1 = 0.05 i2 = 0.10 target1 = 0.01 target2 = 0.99 alpha = 0.5 #學習速率 numIter = 10000 #迭代次數 for i in range(numIter): #正向傳播 neth1 = i1*weight[1-1] + i2*weight[2-1] + bias[0] neth2 = i1*weight[3-1] + i2*weight[4-1] + bias[0] outh1 = sigmoid(neth1) outh2 = sigmoid(neth2) neto1 = outh1*weight[5-1] + outh2*weight[6-1] + bias[1] neto2 = outh2*weight[7-1] + outh2*weight[8-1] + bias[1] outo1 = sigmoid(neto1) outo2 = sigmoid(neto2) print(str(i) + ', target1 : ' + str(target1-outo1) + ', target2 : ' + str(target2-outo2)) if i == numIter-1: print('lastst result : ' + str(outo1) + ' ' + str(outo2)) #反向傳播 #計算w5-w8(輸出層權重)的誤差 pdEOuto1 = - (target1 - outo1) pdOuto1Neto1 = sigmoidDerivationx(outo1) pdNeto1W5 = outh1 pdEW5 = pdEOuto1 * pdOuto1Neto1 * pdNeto1W5 pdNeto1W6 = outh2 pdEW6 = pdEOuto1 * pdOuto1Neto1 * pdNeto1W6 pdEOuto2 = - (target2 - outo2) pdOuto2Neto2 = sigmoidDerivationx(outo2) pdNeto1W7 = outh1 pdEW7 = pdEOuto2 * pdOuto2Neto2 * pdNeto1W7 pdNeto1W8 = outh2 pdEW8 = pdEOuto2 * pdOuto2Neto2 * pdNeto1W8 # 計算w1-w4(輸出層權重)的誤差 pdEOuto1 = - (target1 - outo1) #之前算過 pdEOuto2 = - (target2 - outo2) #之前算過 pdOuto1Neto1 = sigmoidDerivationx(outo1) #之前算過 pdOuto2Neto2 = sigmoidDerivationx(outo2) #之前算過 pdNeto1Outh1 = weight[5-1] pdNeto2Outh2 = weight[7-1] pdEOuth1 = pdEOuto1 * pdOuto1Neto1 * pdNeto1Outh1 + pdEOuto2 * pdOuto2Neto2 * pdNeto1Outh1 pdOuth1Neth1 = sigmoidDerivationx(outh1) pdNeth1W1 = i1 pdNeth1W2 = i2 pdEW1 = pdEOuth1 * pdOuth1Neth1 * pdNeth1W1 pdEW2 = pdEOuth1 * pdOuth1Neth1 * pdNeth1W2 pdNeto1Outh2 = weight[6-1] pdNeto2Outh2 = weight[8-1] pdOuth2Neth2 = sigmoidDerivationx(outh2) pdNeth2W3 = i1 pdNeth2W4 = i2 pdEOuth2 = pdEOuto1 * pdOuto1Neto1 * pdNeto1Outh2 + pdEOuto2 * pdOuto2Neto2 * pdNeto2Outh2 pdEW3 = pdEOuth2 * pdOuth2Neth2 * pdNeth2W3 pdEW4 = pdEOuth2 * pdOuth2Neth2 * pdNeth2W4 #權重更新 weight[1-1] = weight[1-1] - alpha * pdEW1 weight[2-1] = weight[2-1] - alpha * pdEW2 weight[3-1] = weight[3-1] - alpha * pdEW3 weight[4-1] = weight[4-1] - alpha * pdEW4 weight[5-1] = weight[5-1] - alpha * pdEW5 weight[6-1] = weight[6-1] - alpha * pdEW6 weight[7-1] = weight[7-1] - alpha * pdEW7 weight[8-1] = weight[8-1] - alpha * pdEW8 # print(weight[1-1]) # print(weight[2-1]) # print(weight[3-1]) # print(weight[4-1]) # print(weight[5-1]) # print(weight[6-1]) # print(weight[7-1]) # print(weight[8-1])
到此這篇關于python里反向傳播算法詳解的文章就介紹到這了,更多相關python里反向傳播算法是什么內容請搜索好吧啦網以前的文章或繼續瀏覽下面的相關文章希望大家以后多多支持好吧啦網!
相關文章:
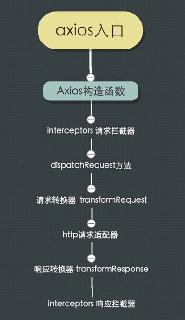